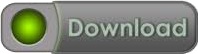
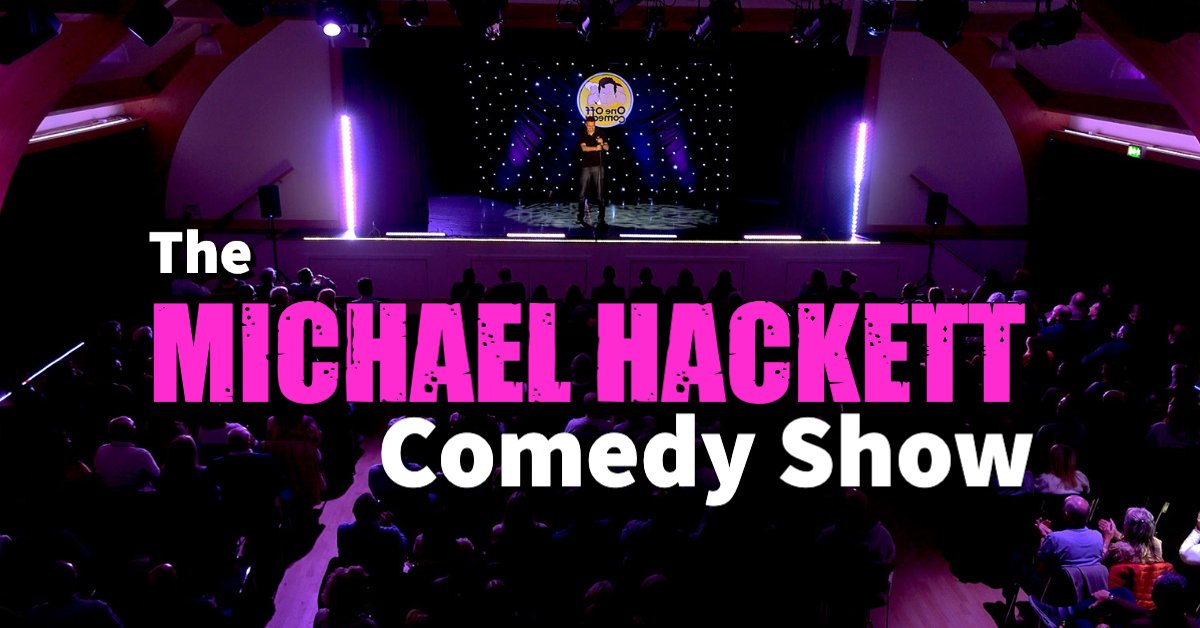
Results favor the use of visual information to improve the accuracy of audio-based methods, as well as for being applied without audio-signal help. Four different Data Fusion techniques were evaluated: feature fusion, sum rule, product rule and an approach in which the visual information is used as prior distribution, which resembles the way humans recognize chords being played by a guitarist. Experiments were conducted regarding classification accuracy comparisons among methods using audio, video and the combination of the two signals. The visual descriptor consists of the rough position of the fingertips in the guitar fretboard, found by using special markers attached to the middle phalanges and fiducials attached to the guitar body. We developed a video-based method for chord recognition which is analogous to the state-of-the-art audio-based counterpart, relying on a Supervised Machine Learning algorithm applied to a visual chord descriptor. In the first part, we discuss the use of visual information for the tasks of recognizing notes and chords. From the point of view of synthesis, visual properties of the guitar fretboard are taken into account in the development of bi-dimensional interfaces for music performance, improvisation, and automatic composition. From the analysis point of view, the use of a video camera for human-computer interaction in the context of a user playing guitar is studied. In this work we explore the visual interface of the guitar.
#Tux guitar hold note over multiple measures update
Since not all possible varying patterns of the data used in our work are available, an online learning approach is applied to efficiently update the original model based on the new data added to the training dataset. In addition, a new dataset for visual transcription of piano music is created and made available to researchers in this area.
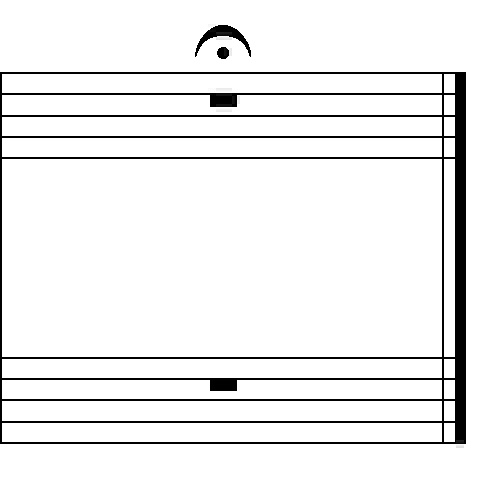
The proposed system has a low latency (about 20 ms) in real-time music transcription. A high accuracy with an average F1 score of 0.95 even under non-ideal camera view, hand coverage, and lighting conditions is achieved. The whole process in this technique is based on visual analysis of the piano keyboard and the pianist’s hands and fingers. In this paper, a new real-time learning-based system for visually transcribing piano music using the CNN-SVM classification of the pressed black and white keys is presented. In order to deal with the challenges arising from acoustic-based music information retrieval such as automatic music transcription, the video of the musical performances can be utilized.
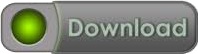